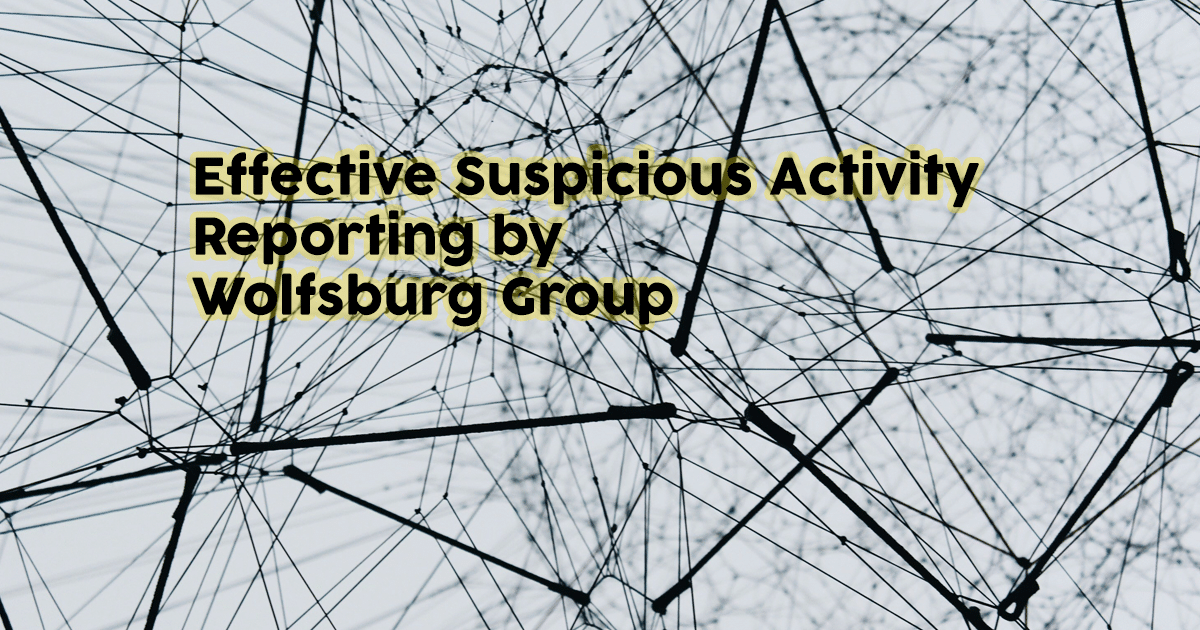
The Wolfsberg Group’s statement on effective monitoring of suspicious activity highlights the evolving strategies financial institutions (FIs) should adopt to combat financial crime was released on 1st July 2024. The statement underscores the importance of transitioning from traditional methods to more sophisticated, technology-driven approaches, particularly through the use of machine learning (ML) and artificial intelligence (AI). Here, we explore the most compelling points of the Wolfsberg Group’s recommendations and tie them to the advancements in ML.
Key Points from the Wolfsberg Group’s Statement
1. Focus on Risk-Based Monitoring: The Wolfsberg Group emphasizes the shift from a prescriptive, rules-based approach to a risk-based approach (RBA). This transition aims to prioritize quality over quantity, ensuring that FIs allocate their resources towards monitoring activities that genuinely mitigate financial crime risks. Traditional methods often result in a high volume of suspicious activity reports (SARs) with minimal actionable intelligence. By focusing on RBA, FIs can enhance the effectiveness of their monitoring systems, thereby producing more valuable outputs for law enforcement agencies.
2. Enhancing Analytical Capabilities: To support the RBA, FIs are encouraged to develop robust analytical capabilities. This involves integrating ongoing customer due diligence (CDD) with transactional monitoring to gain a holistic view of customer behavior. The integration of various data sources, including transactional data, customer behavior, and external data, can provide deeper insights into potential suspicious activities.
3. Embracing Technological Innovation: The statement highlights the necessity of leveraging new technologies to improve monitoring systems. Traditional monitoring platforms are often inadequate to address the complexities of modern financial transactions. Advanced technologies, particularly ML and AI, can significantly enhance the detection of suspicious activities by identifying patterns and anomalies that human analysts might miss. These technologies enable FIs to handle large volumes of data more efficiently and accurately.
4. Measuring Effectiveness: A critical aspect of the Wolfsberg Group’s recommendations is the emphasis on measuring the effectiveness of monitoring programs. This involves using metrics that go beyond the volume of SARs filed to assess the quality and usefulness of the information provided. FIs should seek feedback from government authorities on the utility of their reports to refine their monitoring strategies continuously.
Integration with Machine Learning
1. Improved Detection through Machine Learning: ML models excel at detecting complex patterns within vast datasets. In the context of monitoring suspicious activity, ML algorithms can analyze transactional data alongside other behavioral indicators to identify potentially illicit activities more accurately. For instance, supervised learning models can be trained on historical SARs to predict future suspicious transactions, while unsupervised learning models can identify new, previously unrecognized patterns of suspicious behavior.
2. Reducing False Positives: One of the significant challenges in traditional monitoring systems is the high rate of false positives, which can overwhelm compliance teams. ML algorithms can help reduce these false positives by continuously learning from past data and refining their predictive capabilities. This allows FIs to focus their resources on genuinely suspicious activities, thereby increasing operational efficiency and effectiveness.
3. Enhancing Customer Segmentation: Dynamic customer segmentation powered by ML can lead to more precise monitoring. Traditional segmentation methods often rely on static attributes, which may not accurately reflect changing customer behaviors. ML techniques can create dynamic segments based on real-time data, allowing for more responsive and targeted monitoring.
4. Feedback Loop Integration: ML systems benefit from incorporating feedback from case investigations and SARs. By analyzing outcomes of past investigations, ML models can improve their accuracy and adapt to emerging threats. This iterative process ensures that monitoring systems remain effective in identifying new types of financial crime.
Conclusion
The Wolfsberg Group’s statement on effective monitoring of suspicious activity marks a significant shift towards a more sophisticated, technology-driven approach in combating financial crime. By adopting risk-based monitoring, enhancing analytical capabilities, embracing technological innovations like machine learning, and focusing on the effectiveness of monitoring programs, financial institutions can significantly improve their ability to detect and prevent illicit activities. The integration of ML and AI into monitoring systems not only enhances the detection capabilities but also ensures that the fight against financial crime remains agile and effective in the face of evolving threats.
Leave a Reply